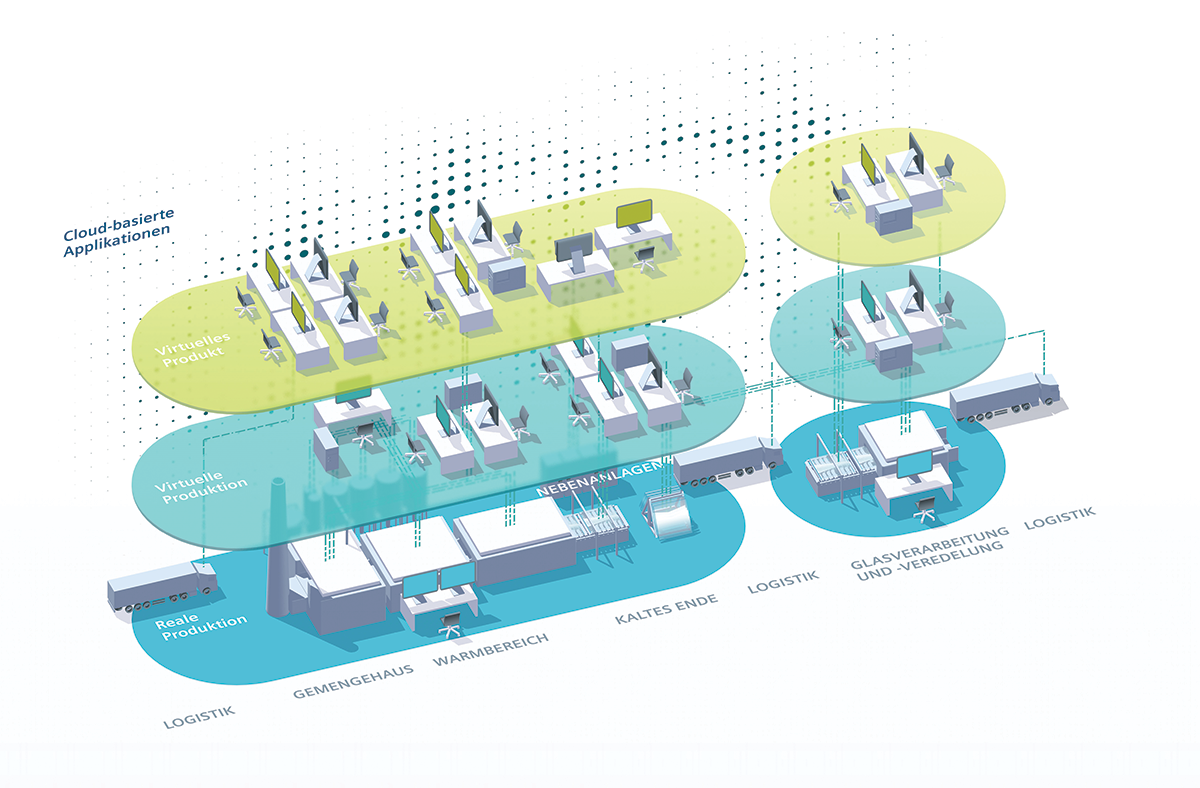
Above: Graphic from Siemens AG demonstrates how digitalization promotes production of glass with transparency at every level.
As digitalization and automation progress, the integration of artificial intelligence is an important step for the flat glass industry to optimize processes, reduce energy consumption and CO2 emissions, and increase international competitiveness. When analyzing errors in manufacturing processes, using AI and deep learning technologies makes for rapid and precise analysis and interpretation of increasingly complex, extensive datasets. The objective: fewer defects and less downtime, producing the same product in less time while using less energy and resources.
The theme “AI in machine technology” is also an important topic at glasstec 2024, the leading trade fair for not only glass producers but also for the machine manufacturers involved. The following are key takeaways about the use of AI in the industry, based on interviews with leaders from VDMA’s Glass Technology Forum and glasstec exhibitors.
Systems that learn and adapt
AI denotes the ability of a machine or system to perform tasks that would require human intelligence, such as learning and problem solving, language recognition and reproduction, image recognition, and possibly “intuition” from experience. “It is the adaptability of machinery and equipment builders that drives innovations and allows our industry to remain competitive,” says Gesine Bergmann, head of the VDMA Glass Technology Forum.
New levels of optimization
“The industry and modern factories are already very advanced, and the efficiency of glass production and the resulting glass quality using conventional means have almost reached the limits of optimization,” says Peter Seidl, head of product management at machinery producer Grenzebach. AI provides companies with new avenues for adding efficiency along production lines.
Florian Sterzing, R&D team leader of data analytics, ISRA Surface Vision, adds, “Initially, these systems learn by given data and then also from each experience acquired. Specialized decision-making AIs can be trained for every application, every production area that generates data. This helps to cut maintenance times, avoid downtimes and, hence, also reduce CO2 emissions: the same product, made in less time with less resources and energy used.”
Predictive analysis
AI systems can be trained to provide predictive analysis and even decision making. “The most important and most heatedly disputed development area currently is predictive analytics, where
AI-assisted systems analyze historical trends, identify patterns and anticipate bottlenecks and inefficiencies, thereby prompting continuous process improvements,” says Sterzing.
Ethical implications of disruptive technologies
Every disruptive technology comes with ethical implications: railways, automobiles, the Internet, mobile communication—wherever new technologies emerge there is always also uncertainty as to whether familiar structures will fall by the wayside. It therefore seems essential that AI systems are developed and used in a fair, transparent and ethically responsible manner while also gauging the implications for the world of work at an early stage, with a view to reshaping it with farsightedness and care in the interest of man.